A blog of the Science and Technology Innovation Program
Prediction and Modeling in a Crisis: The Opportunities for Advancement that COVID Revealed
Novel pathogens present novel challenges to containment and the mitigation of disease spread. After a deluge of COVID-related predictive models, subsequent corrections, and more variants on the way, the push to institute a more transparent and centralized modeling infrastructure in the US and around the world has reached a new level of urgency.
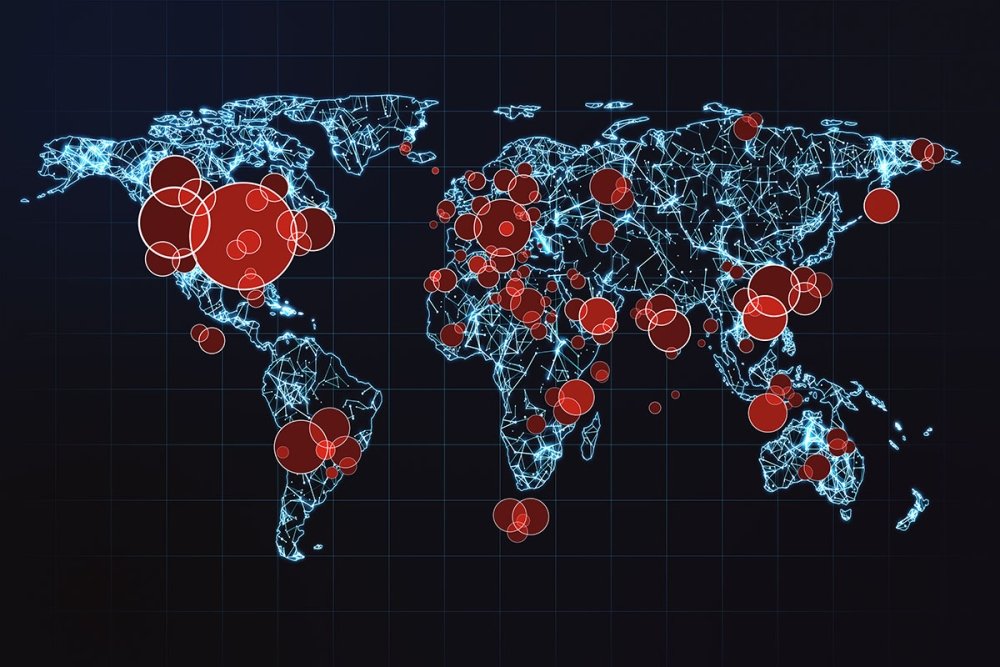
Digital world map with hearts of spread COVID-19 virus by red spots. 3D rendering / Shutterstock
In ideal situations, policymaking derives its power from educated assumptions and projections reported by trusted subject matter experts and acted upon by the policymaking community. However, the data and the subsequent models are rarely a dominant story in national news. Prior to COVID-19, a notable exception to this is the use of predictive modeling, statistical methods to infer future events or outcomes, for climate change mitigation. Climate models are a core feature of the media coverage of our warming earth and have only gained in popularity as climate change has become one of the most pressing policy issues of our generation.
In the past decade, the Intergovernmental Panel on Climate Change’s (IPCC) reports have asserted their preeminence in the field, gaining extensive coverage by major media outlets. This coverage frequently focuses on the findings from the multitude of predictive models included in each of the five IPCC reports. These models work as a call to action for world leaders and give the public a peek into the possible futures that are tied to actions taken today to curb rising temperatures by varying and specific degrees.
For context, the image on page 1037 of the 2018 IPCC report shows predictions for four separate degree-bound scenarios—two of which, RCP8.5 and RCP2.6, are displayed within the figure and further depicted on the right while the other two, RCP4.5 (light blue), RCP6.0 (orange).
To make predictions, climate researchers and data scientists collate data on many factors, including historic trends in temperature increases, CO2 emissions, urbanization, and even natural events like solar variability and volcanoes. Because of its rigorous standards for conducting meta-analyses on the most relevant climate literature and research and its global acclaim, the IPCC is considered the authoritative source on climate models. A single authoritative source allows for a more linear discussion on setting national targets for carbon emissions like those pledged at this past COP 26. Of course, these “linear discussions” are only about the validity of the models, not the negotiations about what to do with them.
Predictive Modeling and COVID-19
Predictive modeling or predictive analysis is a practice that uses past and present data to project future scenarios either through SEIR models or agent-based models. Machine learning and artificial intelligence take these models a step forward and “learn” from the predictive models by making new assumptions, testing those assumptions, and then learning from those tests, autonomously. For the purposes of this article, it’s helpful to understand that the term model is sometimes used as a catch-all for forecasting done using just predictive analysis as well as more complex machine learning.
The climate models serve as a critical example of how decision-makers use predictive modeling for high-stakes policy intervention. Climate negotiations and debates hinge on these projections; they also fuel climate activism globally by providing new information to activists, grassroots organizations, and politicians at all levels. Similarly, COVID-19 provided another instance where the need for prediction was not only critical to policy-making—but also was a matter of life and death.
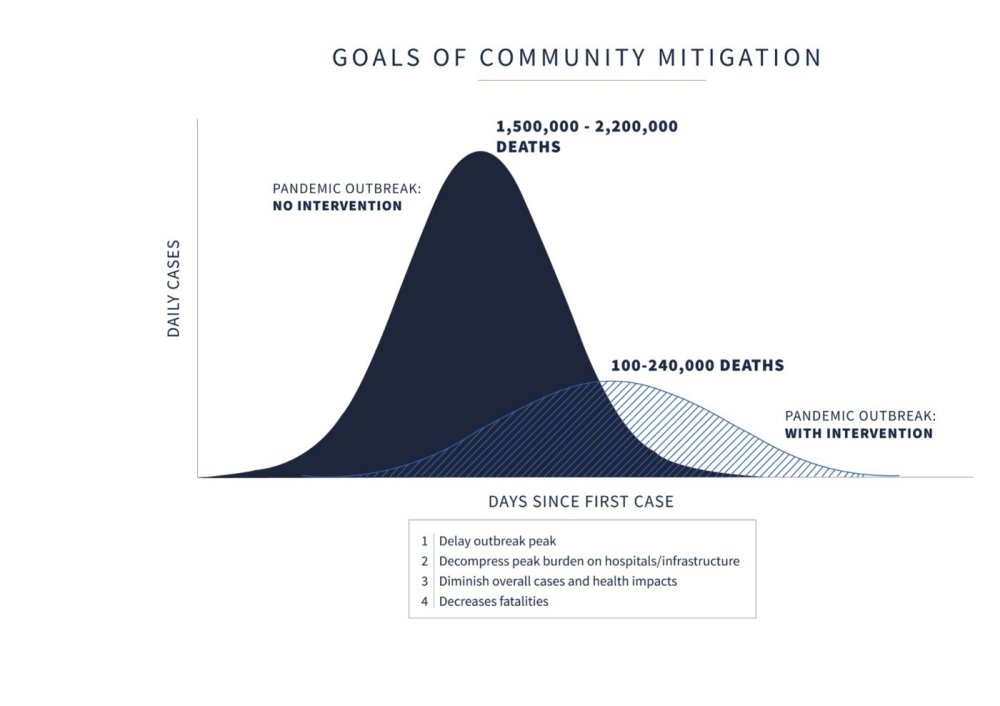
“Goals of Community Mitigation” by United States is licensed under CC BY 3.0 US
Above is a slide from one of the early White House coronavirus briefings. Many recall this slide as a turning point that sparked headlines and social media campaigns to “flatten the curve,” and inspired policies to provide the “intervention” the figure alludes to. This model was actually the sum of seven different models. Most notable among them was the model from the Imperial College London (ICL) which asserted that 2.2 million people would die in the U.S. if there was no action taken by the government to mitigate COVID-19’s spread. ICL’s model is credited by the New York Times with the first round of comprehensive federal recommendations after it was published on March 16, 2020.
Quite quickly, COVID-19 models and projections became a more popular thing for the general public to share, talk about, and base decisions on—not only at the federal level but at the behavioral level as well. Unlike the centralized IPCC-produced models, many different figures from some of the most internationally respected institutions flooded Twitter feeds, cable news, and print media. FiveThirtyEight.com and the COVID-19 Forecasting Hub compiled some of the most trusted forecasts to show the differences between them.
The journalists associated with the project were right to point out that “looking at multiple models is better than looking at just one because it's difficult to know which model will match reality the closest.” But the models themselves and the number of models that were ostensibly informing local decision-making caused some confusion. This was especially true surrounding the question: What is the nation’s most authoritative projection?
Models from the Institute for Health Metrics and Evaluation (IHME) were held up as the gold standard and used by the White House. In parallel, other modeling groups out of Columbia University and the University of Pennsylvania began to influence local and state decisions. Like the climate modelers, COVID-19 modelers had to choose data and make assumptions about the known and, understandably, unknown factors that could influence disease spread.
Unlike climate change, COVID-19 is a new phenomenon without decades of data and trends. While models existed for diseases like influenza and even other coronaviruses, nothing could provide true clarity in the earliest and most consequential days of the pandemic. Modelers had to consider issues like whether policies would be instituted to keep people home, whether or not people would respect the new policies, how infectious the virus was, how many people one person should expect to come into contact with daily (considering the new policies), among many others.
A widely circulated preprint out of the University of Sydney and Northwestern University discredited early IHME models. This is an example of how even the leaders in the field of prediction were wading into the unknown. Lack of historical data, gaps in real-time data collection, and the generalized chaos of spring 2020 lead to a modeling bubble that some believe burst under pressure. The multitude of models were not created in bad faith but did exacerbate the patchwork nature of the U.S.’s COVID response. The algorithms and models that power the AI used to construct more complex and autonomous models are as biased as the creator(s) choose, consciously or otherwise. Researchers make assumptions concerning what data to include and the weights given to independent variables that affect outcomes. Applying assumptions is a necessary part of the modeling practice, but scrutinizing assumptions is just as necessary.
What Comes Next
Undoubtedly, models like the ones from the Imperial College London and the IHME made positive impacts on the way we started to treat COVID-19 as an immediate threat to human life. The policies these models inspired set into motion future mandates and an understanding that while the risk of COVID is not something that will just go away, it is something that can be controlled with effective government action.
However, the amount of variations and biases at play when forecasting a novel pandemic’s potential makes the modeling process confusing and potentially risky. Moving forward there are ways to leverage the best AI has to offer while taking into account AI models’ limitations. These recommendations are not meant to advise on government intervention in this space but to push the US public health apparatus in a direction of cohesion and openness.
Encourage a more open culture for conducting disease modeling where modelers are more upfront with their data biases, the explanations behind them, and funding streams. By opening up the modeling process conducted by research organizations, more scrutiny will naturally follow, which will reveal the gaps in certain models. An open platform like the pre-print servers bioRxiv and medRxiv would also allow researchers to collaborate and learn from their colleagues’ decisions more quickly.
A centralized authority for forecasting pandemics on a national level, at least. If there were to be an open platform for the modeling community to use there would still be an issue of too many models. With a centralized authority, like the IPCC, tasked with the integration of models, the management of relevant health data in an open resource, and the publication of nationwide models, the playing field could become far more digestible to local governments, businesses, and the public.
Work to shift the cultural understanding of scientific uncertainty. COVID-19 has furthered the dialogue concerning the purpose of science as both an institution and a discipline. As an institution, science is built on its ability to discover, communicate that discovery, and maintain the trust of scientists and non-scientists alike. However, scientists’ professional need to assert their degree of uncertainty can create a sense of confusion during the communication process. Uncertainty is integral to effective science and communicating it to a non-technical audience is integral to effective science policy. Bridging the gap between how scientists understand and express uncertainty and how the public understands and acts on uncertainty will strengthen the behavioral influence of popularized models.
A Project with Potential
As of August 2021, the Center for Forecasting and Outbreak Analytics (CFA) was established as a part of the Centers for Disease Control and Prevention (CDC.) Their mission is to lead the United States’ forecasting practices—but the issues detailed throughout this paper remain as the pandemic rages on. In real-time, the CFA’s ability to overcome these challenges will be closely watched by public health leaders.
Quite recently, the CFA had its first official and public meeting hosted by the White House that served as its official launch. The leadership team members of the CFA are regarded as some of the best in the field and have been stalwarts in pandemic mitigation efforts over the past two years. At the April 19th summit, they boasted a new system divided up into teams dubbed Predict, Inform, and Innovate with an emphasis on effective data collection, clear communication, and extremely intricate network collaboration.
CFA and organizations like the recently created Hub for Pandemic and Epidemic Intelligence at the WHO hopefully can address the above recommendations on national and global levels. However, unlike climate data, procurement of accurate, localized, and usable health data still confounds even the most powerful governing bodies. In the wake of COVID-19, these new modeling and analytics agencies will likely rub up against reform in the global health security systems they report to which could complicate their missions in the short term. While these are of course steps in the right direction, it is an open question whether or not they will succeed in providing authoritative and nuanced models for a more informed public.